Deterrence Through AI-Enabled Detection and Attribution
Throughout the Cold War, deterrence theory served as the guiding concept for much of U.S. strategy. Today, however, many practitioners struggle to turn deterrence theory into an effective strategy. While the theory remains fundamentally sound, operations that are difficult to detect and attribute have challenged efforts to implement it as strategy.[1]The increasing proliferation and power of capabilities enabled by sensors, computers, and artificial intelligence (AI) are creating opportunities for deterrers to better detect and attribute offensive operations and to reduce the challenge of translating deterrence theory into effective strategy.
The increasing proliferation and power of capabilities enabled by sensors, computers, and artificial intelligence are creating opportunities for deterrers to better detect and attribute offensive operations . . .
This essay begins by establishing a theoretical framework. It then shows that operations that challenge detection and attribution undermine the credibility of deterrence efforts. Gray zone operations, hybrid operations, and cyber warfare in particular exploit this vulnerability in deterrence strategy. The essay then shows that machine learning and data fusion offer opportunities to improve detection and attribution, increasing the credibility of deterrence strategies and the relevance of deterrence theory. The essay closes with a brief discussion of how such capabilities could affect escalation dynamics between great powers.
A Challenge to Classic Deterrence Theory and Strategy
In his 1966 work, Arms and Influence, Thomas Schelling defined deterrence as seeking “to prevent from action by fear of consequences.”[2] Patrick Morgan then refined Schelling’s definition by elaborating on the relationship between the actors in a dyad, stating that the “essence of deterrence is that one party prevents another from doing something the first party does not want by threatening to harm the other party seriously if it does.”[3] In their contributions to the study of deterrence, Robert Haffa and others before him argued that to effectively deter a challenger, a deterrer must have sufficient capability, credibility, and communication. Haffa defined capability as “the acquisition and deployment of military forces able to carry out plausible military threats to retaliate in an unacceptable manner or to deny the enemy’s objectives in an unaffordable way.”[4] He also defined credibility as “the declared intent and believable resolve to protect a given interest”[5] and described communication as “relaying to the potential aggressor, in an unmistakable manner, the capability and will to carry out the deterrent threat.”[6]
This taxonomy has more than just semantic value. Separating theory and strategy allows the application of theory to be examined without challenging theory itself. Subdividing deterrence into the components of capability, credibility, and communication allows for simplified, useful analysis of how detection and attribution affect the credibility component of the strategy with less focus on capability and communication.
The Challenge to Deterrence Strategy
Deterrence strategy, and a state’s ability to effectively employ it, is dependent on the state’s ability to demonstrate capability and credibility and to clearly communicate those signals to a potential adversary. Credibility cannot be achieved without effective detection and attribution. Without detection, would-be deterrers are unable to mount an effective strategy of denial or threaten retaliation as part of a strategy of punishment. Without attribution, defense is possible, though punishment cannot be targeted at a specific actor. While detection and attribution are not the only components of credibility, they are essential.
Credibility cannot be achieved without effective detection and attribution.
In the aftermath of the First Gulf War, China and Russia sought to increase their conventional military power, while heightening their emphasis on conflict in the gray zone, adopting new hybrid tactics, and employing information operations.[7] This evolution of warfare has continued to serve U.S. adversaries, enabling them to use force, coercion, and deception to pursue their objectives while reducing the probability of detection and attribution; by extension, they have lowered the probability of a credible threat of effective U.S. defense or retaliation.
Gray Zone and Hybrid Operations
Gray zone and hybrid operations pose a detection and attribution challenge due to their inherent ambiguity. Gray zone operations are a strategic approach using coercive actions in the liminal space between armed conflict and more ordinary diplomatic and economic activity.[8] Hybrid operations are an operational approach that use a combination of capabilities—including diplomatic, economic, and informational tools—to create a psychological and physical advantage.[9] Given that gray zone operations are a strategic approach and that hybrid operations are an operational approach, they are neither equivalent terms nor mutually exclusive. Instead, a state can use a gray zone strategy with or without a hybrid operational approach, and vice versa. Both approaches endeavor to create ambiguity. Because of this shared characteristic, both enable an actor to “evade detection outright or to frustrate intelligence efforts to attribute blame, quantify risk, and inform decisive responses.”[10]
Gray zone and hybrid operations pose a detection and attribution challenge due to their inherent ambiguity.
Several countries around the world have implemented such tactics over the last twenty years. To cite a few examples, Israel has used hybrid operations in its fight against Hezbollah; Russia has done so in its 2014 seizure of Crimea and its all-out invasion of Ukraine since February 2022; and countries like China, Russia, Iran, and North Korea have employed such measures against the United States. Detection and attribution remain a challenge.[11] Jake Harrington and Riley McCabe noted in a 2021 brief about the 2020 U.S. presidential election that Russia used “multiple cut-outs and proxies” to employ agents in Nigeria and Ghana to make unattributed gray zone attacks on the United States as part of a broad campaign of election interference.[12]
One reason deterring such operations is so difficult is that they often involve several distinct proxy actors and frequently span multiple “hazy domains,” including “economic influence, information operations, and other political, diplomatic, or commercial activities that defy easy categorization or clear connection to a known campaign of malign behavior.”[13] When seemingly unrelated or insignificant actions could ultimately be revealed to be part of a broader, more insidious plot, targeted actors are left overwhelmed by action inputs, unable to sift through and distinguish “a significant attack from a false alarm.”[14] This is particularly true for information operations, a favored tool of practitioners of hybrid warfare.
Information Operations
Information operations, including cyber operations, offer particularly adept methods of conducting warfare beyond what the United States can easily detect and attribute, and they are widely used in both gray zone and hybrid operations. They can be defined as the “use of social media and other outlets, in addition to traditional efforts, to bolster the narrative of the state through propaganda and to sow doubt, dissent, and disinformation in foreign countries.” Information operations include the use of propaganda (perhaps misleading, but factually true, information), misinformation (unintentionally false information), and disinformation (intentionally false information).[15] Several U.S. adversaries have favored information operations while striving to obtain a strategic advantage over the United States.[16]
According to the Center for Strategic and International Studies (CSIS), since 2006, there have been roughly 1,000 known significant cyber incidents worldwide against “government agencies” or “defense and high tech companies,” or “cyber economic crimes with losses of more than a million dollars.”[17] It is reasonable to expect that many more attacks in this realm have gone unreported, undetected, and unattributed. After all, a significant number of those listed by CSIS remain publicly unattributed to any state or nonstate actor. Many more future cyber incidents will certainly follow.
Russia has come to depend on information operations as its “most effective gray zone tactic”—a tactic that continues “to be well-funded, relentless, and prolific,”[18] as expressed by Moscow’s military doctrine, which scholars have found “frequently equates the strategic impact of information weapons with that of weapons of mass destruction.”[19] Though Russia was responsible for a higher percentage of known information operations using disinformation between 2013 and 2019 than any other country (72 percent), China has also begun to use disinformation operations more. In 2020, Beijing conducted a misinformation campaign on Facebook to promote pro-China messaging in the Philippines.[20] Using cyber operations to not only conduct “espionage and intelligence gathering but also to target other states’ critical infrastructure and disrupt political processes abroad,”[21] Russia and China have demonstrated the power and potential of this method of warfare.[22]
The indicators of an information or cyber operation are typically more difficult, labor intensive, and time-consuming to detect or attribute than those of conventional war.[23] The link between the attack and attacker is typically apparent during most conventional attacks, such as an observed aerial bombing.[24] In contrast, information operations, like those examined by the U.S. cybersecurity firm CrowdStrike, require significant resources to get from detecting an attack by an individual operator to attributing that attack to a specific aggressor. CrowdStrike’s 2014 Putter Panda report outlined one such example in great detail, attributing a series of “coordinated breaches” from a single user handle to a specific individual affiliated with the People’s Liberation Army.[25]
The internet is such that virtual anonymity is possible in the absence of intense scrutiny. According to one expert, “adversaries can exploit any number of system or protocol vulnerabilities to hide or spoof their location and can operate from nearly any physical location.”[26] What is an already difficult knot to untie is further tangled in legal and political complications that delay attribution, especially in circumstances where multilateral cooperation is required.[27] Finally, the mass quantities of data a targeted state might possess on such attacks is only as strong as the targeted state’s ability to understand it. As one scholar has put it, “intelligence services struggle to interpret data, and the more they collect, the more they face the challenge of separating meaningful information from background noise.”[28] Ultimately, this flood of information is the key to attribution, but without the appropriate tools and methods, it is also the fog that obscures these attacks.
The efficacy of deterrence strategy is dependent on the efficacy of an actor’s detection and attribution efforts. To quote one expert, “you cannot deter unless you can punish and you cannot effectively punish unless you have attribution.”[29] This is because a display of resolve, without the credibility of consistently detecting and attributing attacks, is likely to be dismissed. Therefore, any actor that hopes to combat such attacks and ultimately deter future ones needs to strengthen its ability to detect and attribute such tactics. This is not to say that credibility is the product of detection and attribution alone or that strengthening detection and attribution will, on its own, result in credible deterrence. Instead, as necessary but not sufficient components of deterrence, strengthening detection and attribution will shore up one important weakness in deterrence strategies.
How to Strengthen Detection and Attribution
Strengthening attribution and detection would require a country’s government, especially its military services and intelligence agencies, to accomplish several related objectives. First, given that some of the offensive actions described above are designed to avoid detection, intelligence agencies and military services would need to observe more adversary actions in all five domains (land, sea, air, space, and cyber). Second, they would need to understand which of these events are significant, either as actions in their own right or as indicators of future actions. Third, they would then need to focus more attention on potential significant events and to identify when and how these events take place and who is causing them. Finally, all of this information would need to be pulled together into a coherent narrative that would inform policymakers and military and intelligence leaders.
Technological developments, particularly the growing prevalence of sensors and the power of AI, can help intelligence agencies and militaries perform these demanding tasks.
Technological developments, particularly the growing prevalence of sensors and the power of AI, can help intelligence agencies and militaries perform these demanding tasks. Far more of the planet is observed with greater frequency today. The proliferation of large constellations of commercial satellites has been one notable development. Companies like Skybird have observed militaries, nuclear reactor and centrifuge sites, and missile launches for more than a decade.[30] An increasing number of companies like BlackSky, OneAtlas, ICEYE, and others collect imagery from space ever more frequently and provide imagery, including synthetic aperture radar imagery, to governments.[31] The explosive growth in the use of smart phones and the way they combine location data and photos offers another means of collection, especially when photos are posted on social media sites or messaging apps. Internet users also leave data exhaust online that shows their searching, reading, and other habits.[32] These changes are making it more difficult to avoid detection in every domain, even the most traditionally opaque ones like outer space, under the sea, and cyberspace.[33]
While the large increase in sensors will help militaries and intelligence agencies observe significantly more events, this shift also has the potential to complicate their work rather than automatically strengthening detection or attribution. The sheer volume of data creates one challenge. According to one estimate, there were more than 600 million surveillance cameras in China alone prior to the coronavirus pandemic.[34] Data from thousands of satellites, billions of people and bots on social media, and other information sources have the potential to overwhelm both analysts and decision-makers, particularly during crises.[35] To complicate matters further, much of this information comes from commercial partners or nontraditional data sources like open-source intelligence on social media platforms. Tapping into these alternative sources requires intelligence agencies to partner with commercial entities to purchase their data or analysis and to integrate their work into intelligence assessments.[36]
AI can help analysts understand which events are significant, focus their attention, help them attribute actions, and aid them in presenting usable information to decision-makers.
AI can help analysts understand which events are significant, focus their attention, help them attribute actions, and aid them in presenting usable information to decision-makers.[37] The most common definitions of AI revolve around using machines to perform tasks that usually require human or some other form of intelligence.[38]
The Defense Advanced Research Projects Agency sees AI as having come in two broad waves, with another one to come. The first wave involved expert systems that were built manually, often by interviewing subject matter experts and encoding their knowledge as rules, an approach that has proven limited for use at scale.[39] The second and current wave of advances in AI consists mainly of statistical models trained on data via machine learning. While machine learning algorithms, specifically neural networks, have limited contextual capabilities and minimal reasoning abilities, they are quite adept at recognizing patterns in data, especially extremely large data streams when given access to powerful or application-specific hardware. Such an algorithm can discover correlations in such datasets and optimize a computer programming framework to perform assigned tasks. This gives them classification, inference, and prediction capabilities that can be more effective than those of previous generations of software and hardware.
Despite these advances, machine learning has significant limitations. It reflects the limitations and biases of the datasets used to train it. Likewise, the speed at which algorithms can be trained to infer is limited by the computing power available. Such algorithms also struggle to perform in many unstructured environments. Structured environments provide well-defined and discrete parameters, choices, and outcomes. Many of AI’s best known victories, such as AlphaGo’s victory over champion player Lee Se-dol in the board game Go, have taken place in structured environments.[40] When performing tasks in unstructured settings, AI must create and continuously update a model of the world, including potentially unfamiliar environments.[41] Machine learning tools have often struggled to accurately reason in unstructured environments, particularly for the type of high-stakes decision-making performed in warfare.[42] Most machine learning algorithms are also narrow and brittle, performing only a very specific task or set of tasks. Placing such algorithms in unfamiliar conditions, or assigning them unfamiliar tasks, can result in failure, sometimes in unpredictable ways.[43]
The third, still-theoretical wave of AI would be much closer to artificial general intelligence (AGI), or AI that extends beyond narrow capabilities to broader, more human-like abilities.[44] If and when the third wave of AI develops, its systems are expected to perceive, learn, abstract, and reason in ways much closer to humans than first- and second-wave AI systems.[45] One example of a military application of third-wave AI would be “a fully autonomous ship that uses algorithms to maneuver in situations it was not specifically trained for (such as inclement weather or contested waters); it would be capable of planning, relaying, and carrying out military missions similar to the way a human would.”[46] The consensus is that third wave models will take some time to develop. As a result, second-wave machine learning applications will dominate AI-enabled systems for the foreseeable future.[47]
Machine learning offers multiple ways to help collect and process information in a manner that may strengthen attribution and detection efforts. AI-enabled autonomy can help direct intelligence collection in both physical and digital domains by both directly guiding collection assets, such as semi-autonomous drones,[48] and by providing collection guidance called autonomous tipping and cueing for individual assets.[49] Machine learning can also assist with analyzing collected data. Supervised learning algorithms trained for image recognition can identify objects far faster than human analysts can, as in the case of the Department of Defense’s most prominent AI use case, Project Maven.[50] Unsupervised learning algorithms can zero in on patterns in data, including patterns too complicated for humans to identify without algorithmic assistance.[51]
Far from a hypothetical scenario, the U.S. military, intelligence community, and private sector already use AI in ways that can strengthen detection and attribution. The most prominent example is Project Maven, which uses machine vision to help analysts identify objects in footage and imagery much more quickly, and in much greater quantities, than would be possible for human analysts alone. The Army has built on Project Maven’s work to develop Scarlet Dragon, a “target recognition” software that is already used in live fire drills. Prometheus similarly uses AI to sense and identify targets from space.[52] The Air Force used an AI tool developed in just five weeks to pilot a U-2 aircraft in December 2020, has integrated AI into its all-domain operating picture (known as the Advanced Battle Management System), and is partnering with the Space Force to develop an AI-enabled space awareness capability.[53] In the private sector, commercial drones have the ability to autonomously recognize and track specific individuals while developing a real world map, planning a path, and avoiding obstacles.[54]
As a result, intelligence agencies and militaries that integrate AI into their operations and processes have the potential to significantly increase their abilities to collect data, make sense of that information, and highlight important findings for decision-makers. Increasing the collection and visibility of relevant information will strengthen these government actors’ ability to detect activities that previously evaded sensors, were lost in the noise, or that can only be detected by combining multiple data inputs. Doing so will also strengthen their abilities to attribute actions to the relevant parties by associating disparate attribution indicators even in cases involving hard-to-detect activities.[55]
One of the most promising ways to use AI to strengthen detection and attribution is data fusion. Data fusion plays an important role in helping intelligence analysts and military personnel detect and draw connections between phenomena from different types of sensors and in different databases.[56] Drawing on digital signal processing, statistics, control theory, and other fields, militaries have used data fusion for decades to improve intelligence collection and command and control, primarily by combining data streams from the same type of sensors.[57]
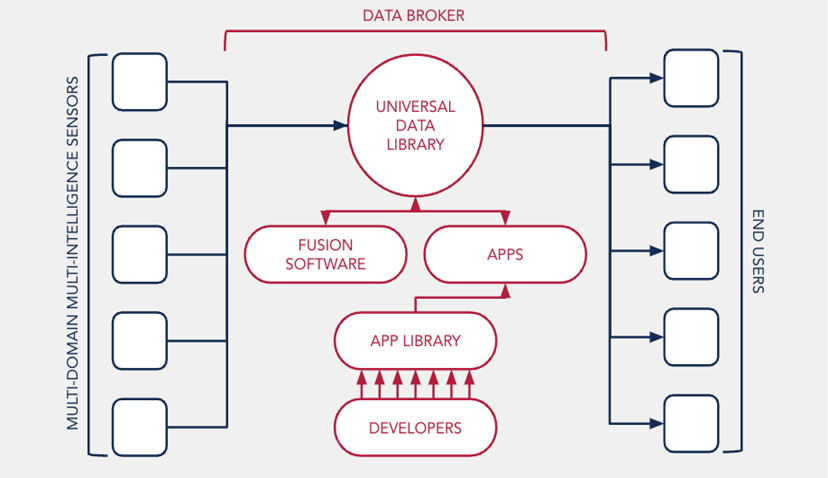
Figure 1. A Data Fusion Model
Data fusion is likely to evolve to be able to fuse many heterogeneous data streams coming from tactical intelligence assets into strategic intelligence analysis.
The growing ubiquity of sensors and the power of AI-enabled analytics means that data fusion is likely to evolve to be able to fuse many heterogeneous data streams coming from tactical intelligence assets into strategic intelligence analysis. Figure 1 shows how a networked system can integrate data from many diverse sensors into usable information for a variety of end users.[58] Together, data fusion systems enable sensors, a data broker, applications, and end users to compile large amounts of data from diverse sources and make it far easier for end users to detect and attribute adversaries’ activities by identifying important information and connecting disparate activities.
- Sensors: Multidomain sensors from multiple intelligence sources provide inputs to the data broker. The sensors include traditional intelligence sources, such as satellite imagery, human intelligence reports, signals intelligence, and electronic intelligence. This would also include publicly available information, such as information posted on social media and commercially available intelligence, such as commercial satellite imagery.[59] As data fusion systems become more sophisticated, a growing number and variety of sensors will be able to provide inputs to the same data broker.
- Data Brokers: The data broker is a database that organizes and catalogs the intelligence provided by the sensors in a format and location on the network that allows this information to be used by the applications in the application library.
- Applications and application library: The application library is a set of software tools available to end users to process and analyze data held by the data broker. While some of the applications are AI-enabled, they would use (potentially several) narrow AI applications and would be directed by a human end user.
- End users: The information produced by applications used to access the fused datasets would support a range of parties including analysts serving in strategic intelligence roles, analysts in operational and tactical military headquarters, fielded units and systems, and even the people and algorithms that guide the sensors that provide such inputs.
Governments that establish a strategic-level data fusion system will be able to improve their ability to detect and attribute adversaries’ activities. Large volumes of information from heterogeneous datasets would be accessible at a single point for analysis with a prepared and regularly updated set of applications. This would allow end users to use many data points to detect otherwise invisible events like cyber attacks or geopolitically motivated financial moves. It would also help end users attribute actions by quickly using a wealth of data to more effectively determine the actors involved, their methods, and who employed them.
Strengthening Deterrence
When an adversary manages to ensure that its operations and preparations for those operations avoid detection and attribution, that reduces the probability that targeted countries will adequately prepare to respond. This increases the probability of the aggressor accomplishing its goals and decreases its likely cost of victory, undermining the credibility of deterrence strategies. Technology is granting intelligence agencies and militaries the ability to monitor an increasingly large portion of the world using an ever greater variety of sensors, to combine that intelligence into a more coherent whole, and to understand it using AI-enabled software.[60] As this shift takes place, governments will be significantly more likely to be able to detect and quickly attribute gray zone and hybrid tactics, cyber incursions, and other types of attacks.
Strengthening detection and attribution would create more opportunities to try to defeat or deter gray zone and hybrid tactics, cyber attacks, and other operations by adversaries.
Strengthening detection and attribution would create more opportunities to try to defeat or deter gray zone and hybrid tactics, cyber attacks, and other operations by adversaries. Detecting preparations for operations or operations themselves would allow targeted governments to plan and prepare specific diplomatic, military, or economic responses to reduce the probability of an enemy’s success or increase the cost of the foe’s offensive operations. For example, if Ukraine had been able to detect Russia’s preparations to send irregular forces into Crimea in 2014, or their arrival in the region prior to more conventional Russian operations, they would have been better equipped to stage forces in Crimea, preempt Russian information operations, and strengthen Ukrainian cyber defenses, reducing the probability of a successful Russian seizure of Crimea or increasing the cost of doing so. The United States and the North Atlantic Treaty Organization (NATO) did detect Russian preparations for an invasion of Ukraine in 2021 and 2022, and they consequently helped disrupt Russian information and cyber operations.[61] The United States and NATO did not, however, deter a conventional attack, reinforcing the point that detection and attribution merely create opportunities to defeat or deter attacks and do not automatically result in deterrence success.
Retroactive attribution also can possibly strengthen efforts to deter operations that have typically been difficult to attribute. More quickly attributing the identities and intent of offensive actors in cyberspace, of state-affiliated criminals, or of geopolitical actions whose moves are disguised as benign economic activity such as some infrastructure projects or dual-use research would help the identifying countries coordinate responses with their allies and partners. While this might result in military action, it might also help partners coordinate sanctions, increasing the economic cost of adversarial offensive operations.
The emergence of machine learning and its application through a data broker model of data fusion has the potential to strengthen deterrence. As these technologies continue to improve, states will probably come to believe that their adversaries are highly likely to detect and attribute their preparations for or execution of offensive operations, and targeted countries could therefore have greater opportunities to punish, or even block, offensive operations. Stephen Van Evera’s research in Causes of War showed that states that believe they do not have a first-mover advantage, including because they cannot achieve the element of surprise, are less likely to engage in offensive operations.[62] As John Mearsheimer has argued, “deterrence—a function of the costs and risks associated with military action—is most likely to obtain when an attacker believes that his probability of success is low and that the attendant costs will be high.”[63] Decreasing the probability of cheap or quick victories has the potential to strengthen deterrence and stabilize relationships between competing great powers.[64]
Adversaries’ Reactions to Greater Detection Could Prove Destabilizing
It is also possible that even though improving detection and attribution would strengthen traditional forms of deterrence, such a strategy would also prove to be destabilizing. States that are politically committed to outcomes that can only be achieved through military operations may shift from relying on ambiguity to more overt operations designed to reduce their adversaries’ time to react to their own military mobilization or to mitigate the effectiveness of a targeted country’s reactions. Some examples of more overt kinds of operations an adversary could employ include:
- Maintaining a highly mobilized, offensive force posture: To reduce the risk of having another country detect their mobilization efforts, states may rely on maintaining their forces at a high degree of readiness for offensive operations. The Russian military staged training exercises near Ukraine’s border prior to invading to ensure its forces were prepared to invade with minimal changes to their logistics or posture.[65]
- Striving to mobilize troops more rapidly than an opponent: To reduce the time a targeted country has to react to the detection of an adversary’s mobilization efforts, states may focus on mobilizing more quickly than their foe. The most famous example of this technique comes from the buildup to World War I, when several nations—particularly France, Germany, and Russia—focused on their ability to mobilize their armies more quickly than their adversaries.[66]
- Attempting disruptive attacks: Rather than trying to decrease the time a targeted country has to react to offensive operations, states could focus on reducing their ability to react. Such efforts could include cohesion attacks that sow societal discord to focus political leaders’ attention on domestic issues,[67] attacks on infrastructure for preventing military mobilization, or even first strikes of the type envisioned during the Cold War.
Each of these strategies would have destabilizing effects. Moving militaries closer to being fully mobilized for war, either through a very high state of readiness or preparations to mobilize, has the potential to start an escalatory cycle with potential adversaries. Similarly, disruptive attacks had a robust history of destabilizing effects during the early nineteenth century and again during the Cold War.[68] The same could prove true today.
Conclusion
Seeking to remedy a country’s detection and attribution capabilities through improved sensing, data fusion, and AI does not guarantee any level of global stability. It only offers the opportunity for a credible deterrence strategy. The effects of such a strategy could increase or decrease stability depending on the intent of the actors involved, how adeptly the strategy is applied, and how well it suits the needs of the moment. As with any strategy, the odds of deterrence achieving its desired effects are dependent on a clear understanding of the world at a given moment. Techniques that improve a country’s understanding of the world like wargaming and monitoring for specific indicators—like signs that an adversary is relying on a high state of military readiness or planning to employ disruptive attacks—should be used frequently.
Nonetheless, reintroducing a viable deterrence strategy would likely help address the challenge posed by ambiguous gray zone, hybrid, and cyber operations. Regardless of the unknown consequences of such a deterrence strategy, detection and attribution will likely improve in the years ahead.
About the Authors
Justin Lynch is the Senior Director for Defense at the Special Competitive Studies Project (SCSP). Prior to SCSP, he served at the National Security Commission on Artificial Intelligence, at the House Armed Services Committee, and in the United States Army. He is a term member at the Council on Foreign Relations and a nonresident fellow at the Atlantic Council.
Emma Morrison is a national security professional who has served at the Special Competitive Studies Project and at the House Armed Services Committee.
References
[1] Scholars like Patrick Morgan and Reuben Steff differentiate between deterrence theory and strategy. See Patrick Morgan, Deterrence Now (Cambridge, UK: Cambridge University Press, 2003), 10; and Reuben Steff, “Nuclear Deterrence in a New Age of Disruptive Technologies and Great Power Competition,” in Deterrence: Concepts and Approaches for Current and Emerging Threats, ed. Anastasia Filippidou (Oxfordshire, UK: Springer Cham, 2020), 58.
[2] Thomas Schelling, Arms and Influence (New Haven, CT: Yale University Press, 2008), 71.
[3] Morgan, Deterrence Now, 1.
[4] Robert Haffa Jr., “The Future of Conventional Deterrence: Strategies for Great Power Competition,” Strategic Studies Quarterly 12, no. 4 (Winter 2018): 96–97, https://www.jstor.org/stable/26533617.
[5] Ibid.
[6] Ibid.
[7] Rush Doshi, The Long Game: China’s Grand Strategy to Displace American Order (Oxford, UK: Oxford University Press, 2021).
[8] Bonny Lin, et. al, “A New Framework for Understanding and Countering China's Gray Zone Tactics,” RAND Corporation, 2022, 1, https://www.rand.org/pubs/research_briefs/RBA594-1.html.
[9] James K. Wither, “Defining Hybrid Warfare,” Concordian: Journal of European Security and Defense Issues, December 11, 2019, 8, https://perconcordiam.com/defining-hybrid-warfare.
[10] Harrington and McCabe, “Detect and Understand,” 2–3.
[11] Tark Solmaz, “‘Hybrid Warfare’: One Term, Many Meanings,” Small Wars Journal, February 25, 2022, https://smallwarsjournal.com/jrnl/art/hybrid-warfare-one-term-many-meanings; and Kathleen Hicks and Alice Hunt Friend, By Other Means Part I: Campaigning in the Gray Zone (Washington, DC: Center for Strategic and International Studies, 2019), 14.
[12] Harrington and McCabe, “Detect and Understand,” 3.
[13] Ibid., 5.
[14] Therese Delpech, Nuclear Deterrence in the 21st Century: Lessons From the Cold War for a New Era of Strategic Policy (Santa Monica, CA: RAND Corporation, 2012), 154–155.
[15] Catherine A. Theohary, “Defense Primer: Information Operations,” Congressional Research Service, December 9, 2022, https://sgp.fas.org/crs/natsec/IF10771.pdf.
[16] Hicks and Hunt Friend, By Other Means Part I, 7–8.
[17] Center for Strategic and International Studies, “Significant Cyber Incidents Since 2006,” Center for Strategic and International Studies, 2022, https://www.csis.org/programs/strategic-technologies-program/significant-cyber-incidents.
[18] Ibid., 7, 9.
[19] Michelle Grise, et al., Rivalry in the Information Sphere: Russian Concepts of Information Confrontation (Santa Monica, CA: RAND Corporation, 2022), xi.
[20] Azeem Azhar, The Exponential Age: How Accelerating Technology Is Transforming Business, Politics, and Society (New York: Diversion Books, 2021), 225; and Nathaniel Geicher, “Removing Coordinated Inauthentic Behavior,” Facebook (Meta), September 22, 2020, https://about.fb.com/news/2020/09/removing-coordinated-inauthentic-behavior-china-philippines.
[21] Hicks and Hunt Friend, By Other Means Part I, 7–8.
[22] Nicole Perlroth, “How China Transformed into a Prime Cyber Threat to the U.S.,” New York Times, July 20, 2021, https://www.nytimes.com/2021/07/19/technology/china-hacking-us.html.
[23] Timothy M. McKenzie, Is Cyber Deterrence Possible?: Air University Perspectives on Cyber Power (Maxwell Air Force Base: Air University Press, 2017), 7–8; and Thomas Rid and Ben Buchanan, “Attributing Cyber Attacks,” Journal of Strategic Studies 38, no. 1–2 (2015): 32.
[24] Rid and Buchanan, “Attributing Cyber Attacks,” 13.
[25] CrowdStrike Global Intelligence Team, “CrowdStrike Intelligence Report: Putter Panda,” CrowdStrike, 2014, https://cdn0.vox-cdn.com/assets/4589853/crowdstrike-intelligence-report-putter-panda.original.pdf; and Rid and Buchanan, “Attributing Cyber Attacks,” 13.
[26] McKenzie, Is Cyber Deterrence Possible?, 8.
[27] Ibid.
[28] Joshua Rovner, “Cyber War as an Intelligence Contest,” War on the Rocks, September 16, 2019, https://warontherocks.com/2019/09/cyber-war-as-an-intelligence-contest.
[29] Bob Gourley, Towards a Cyber Deterrent, Cyber Studies Conflict Association, May 29, 2008, 1, https://papers.ssrn.com/sol3/papers.cfm?abstract_id=1542565.
[30] Delpech, Nuclear Deterrence in the 21st Century, 146.
[31] Debra Werner, “Earth Imagery Companies Reimagine Satellite Tasking,” Space News, July 12, 2022, https://spacenews.com/satellite-tasking.
[32] Justin Lynch and Emma Morrison, et. al, “The Future of Conflict and New Requirements of Defense” Special Competitive Studies Project, October 2022, 4, https://www.scsp.ai/wp-content/uploads/2022/10/Defense-Panel-IPR.pdf.
[33] Ibid., 11.
[34] Mark Leonard, The Age of Unpeace: How Connectivity Causes Conflict (London: Bantam Press, 2021), 35.
[35] Steff, “Nuclear Deterrence in a New Age of Disruptive Technologies and Great Power Competition,” 70.
[36] Harrington and McCabe, “Detect and Understand,” 4.
[37] Hicks and Hunt Friend, By Other Means Part I, 30.
[38] National Security Commission on Artificial Intelligence: Interim Report (Arlington, VA: National Security Commission on Artificial Intelligence, November 2019), 53, https://www.nscai.gov/wp-content/uploads/2021/01/NSCAI-Interim-Report-for-Congress_201911.pdf.
[39] John Launchbury, “A DARPA Perspective on Artificial Intelligence,” Defense Advanced Research Project Agency, 3–7, https://www.darpa.mil/attachments/AIFull.pdf.
[40] Gary Marcus and Ernest Davis, Rebooting AI: Building Artificial Intelligence We Can Trust (New York: Pantheon Books, 2019), 20–21.
[41] M.L. Cummings, “Artificial Intelligence and the Future of Warfare,” Chatham House, January 2017, 4.
[42] Marcus and Davis, Rebooting AI, 20–22.
[43] Charles Q. Choi, “7 Revealing Ways AIs Fail,” IEEE Spectrum, September 21, 2021, https://spectrum.ieee.org/ai-failures.
[44] National Security Commission on Artificial Intelligence, Final Report (Arlington, VA: National Security Commission on Artificial Intelligence, March 2021), 35–36, https://www.nscai.gov/wp-content/uploads/2021/03/Full-Report-Digital-1.pdf.
[45] Launchbury, “A DARPA Perspective on Artificial Intelligence,” 26–29.
[46] Government Accountability Office, “Artificial Intelligence: Status of Developing and Acquiring Capabilities for Weapon Systems,” February 2022, 5, https://www.gao.gov/assets/gao-22-104765.pdf.
[47] Launchbury, “A DARPA Perspective on Artificial Intelligence,” 26–29.
[48] Michael Horowitz, “Artificial Intelligence, International Competition, and the Balance of Power,” Texas National Security Review 1, no. 3 (2018): 41.
[49] Muhammad Irfan Ali, “Tip and Cue Techniques for Efficient Near Real-Time Satellite Monitoring of Moving Objects,” Iceye, January 28, 2021, https://www.iceye.com/blog/tip-and-cue-technique-for-efficient-near-real-time-satellite-monitoring-of-moving-objects; and “Autonomous Cueing and Tipping of ISR and OPIR Networks Tool (ACTION): Award Information,” Small Business Innovation Research, 2015, https://www.sbir.gov/sbirsearch/detail/824473.
[50] Government Accountability Office, “Artificial Intelligence,” 15.
[51] Marco Iansiti and Karim R. Lakhani, Competing in the Age of AI: Strategy and Leadership When Algorithms and Networks Run the World (Boston, MA: Harvard Business Review Press, 2020), 65–67.
[52] Government Accountability Office, “Artificial Intelligence,” 15–20.
[53] Ibid., 18–20.
[54] Azhar, The Exponential Age, 257.
[55] Hicks and Hunt Friend, By Other Means Part I, vi.
[56] David Hall and James Llinas, “An Introduction to Multisensor Data Fusion,” Proceedings of the IEEE, 85, no. 1 (1997), 6.
[57] Ibid.
[58] The data fusion model and the graph in the text used to depict it is an abstraction based on the authors’ interviews with a number of government and private sector engineers and analysts in May 2022.
[59] Department of Defense, Office of the Undersecretary of Defense, “DoD Directive 3115.18: DoD Access to and Use of Publicly Available Information (PAI),” Department of Defense, Office of the Undersecretary of Defense, June 11, 2019, https://irp.fas.org/doddir/dod/d3115_18.pdf; and Bob Ashley and Neil Wiley, “How the Intelligence Community Can Get Better at Open Source Intel,” DefenseOne, July 16, 2021,
https://www.defenseone.com/ideas/2021/07/intelligence-community-open-source/183789.
[60] Lynch and Morrison, “The Future of Conflict and the New Requirements of Defense,” 10–12.
[61] “Ukraine Tensions: Russia Invasion Could Begin Any Day, US Warns,” BBC, February 12, 2022, https://www.bbc.com/news/world-europe-60355295; “Inside a US Military Cyber Team’s Defence of Ukraine,” BBC, October 30, 2022, https://www.bbc.com/news/uk-63328398; and Nick Beecroft, “Evaluating the International Support to Ukrainian Cyber Defense,” Carnegie Endowment for International Peace, November 3, 2022, https://carnegieendowment.org/2022/11/03/evaluating-international-support-to-ukrainian-cyber-defense-pub-88322.
[62] Stephen Van Evera, Causes of War (Ithaca, NY, Cornell University, 1999), 68–71.
[63] Mearsheimer, Conventional Deterrence, 11.
[64] Ibid., 14–44.
[65] Alexander Marrow and Maria Kiselyova, “Russia, Holding Military Drills Near Ukraine, Says Ties With U.S. ‘on the Floor,’” Reuters, February 14, 2022, https://www.reuters.com/world/europe/russia-amid-ongoing-drills-near-ukraine-says-ties-with-us-are-on-floor-2022-02-14.
[66] Marc Trachtenberg, “The Meaning of Mobilization in 1914,” International Security 15, no. 3 (1990): 120–150, https://muse.jhu.edu/pub/6/article/447259/summary.
[67] Julia Ioffe, “The History of Russian Involvement in America’s Race Wars,” Atlantic, October 21, 2017, https://www.theatlantic.com/international/archive/2017/10/russia-facebook-race/542796.
[68] Morgan, Deterrence Now, 6–7.